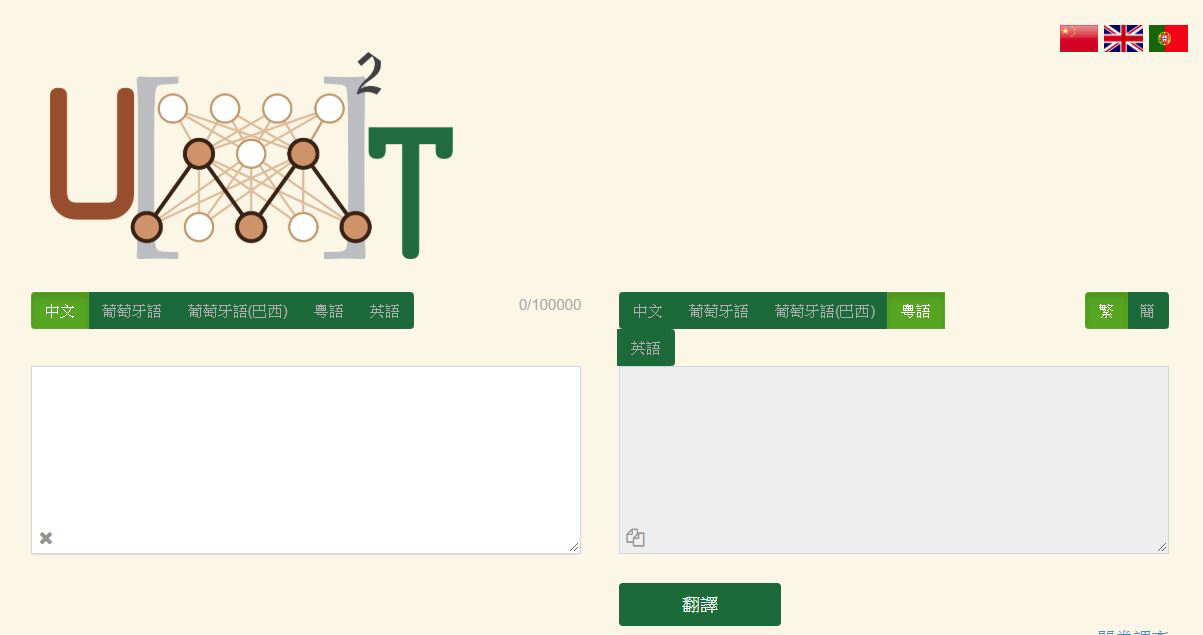
The University of Macau (UM) has achieved yet another breakthrough in machine translation! A research team from UM recently developed a new system for translation between Cantonese and Mandarin. The system can efficiently and accurately translate text between the two dialects. Already available on the internet, the translation system is significant for the integration and development of the economic, cultural, and tourism industries in the Guangdong-Hong Kong-Macao Greater Bay Area.
The Cantonese-Mandarin translation system was developed by UM’s Natural Language Processing & Portuguese – Chinese Machine Translation Laboratory (NLP2CT). As a response to China’s national development strategies, examples of which include the Belt and Road Initiative and the Greater Bay Area, the lab initiated a research study titled ‘Unsupervised Neural Dialect Translation with Commonality and Diversity Modeling’ by utilising Macao’s unique economic and cultural advantages. The related paper was accepted at the Association for the Advancement of Artificial Intelligence (AAAI) Conference on Artificial Intelligence 2020, a top international conference in the field. This year’s event received a record number of papers (8,800), and only 1,591 were accepted. The Cantonese-Mandarin translation system is now available on the internet. Currently, the UM research team is developing a machine translation system and a simultaneous interpretation system between Cantonese and Portuguese, which will use the world’s latest technologies to improve trilingual machine translation among Chinese, Portuguese, and English. They will represent new breakthroughs after the UM-CAT, an online Chinese-Portuguese-English computer-aided translation platform.
Cantonese-Mandarin translation belongs to the category of dialect translation. Although the two dialects bear some similarities, there are differences in syntax. With a significant lack of parallel data between the two dialects, it is difficult to develop an ideal translation model based on current machine translation methods. For this reason, the research group from UM proposed a novel Unsupervised Neural Machine Translation (UNMT) model by introducing Pivot-Private embeddings and coordinating the learning of word representations from both the encoder and decoder in a layer-wise approach, to model the commonalities and diversities of Cantonese and Mandarin at different levels (morphology, syntax, and semantics). The model achieved state-of-the-art Cantonese-Mandarin translation performance only by using monolingual data, significantly outperforming the rule-based approaches and the existing unsupervised NMT models. This approach can greatly enhance translation quality and both machine self-evaluation and human evaluation have confirmed the new approach’s high degree of efficiency and accuracy.
The multidisciplinary team at the lab has won numerous awards for their achievements, including a second prize at the Macao Science and Technology Awards in the Science and Technology Progress Award category for their project that studied the technologies related to Chinese/Portuguese machine translation systems and the applications of the systems. The neural-based machine translation systems developed by the lab won several prizes at the constraint English-to-Chinese machine translation campaign organised under the 13thChina Workshop on Machine Translation (CWMT 2017). In the future, the lab hopes to achieve new breakthroughs in the area of machine translation in order to remove language barriers between Guangdong, Hong Kong, Macao, and Portugal, and to fulfill the need for ever-increasing cultural exchange between Macao, the Greater Bay Area, and Portuguese-speaking countries.