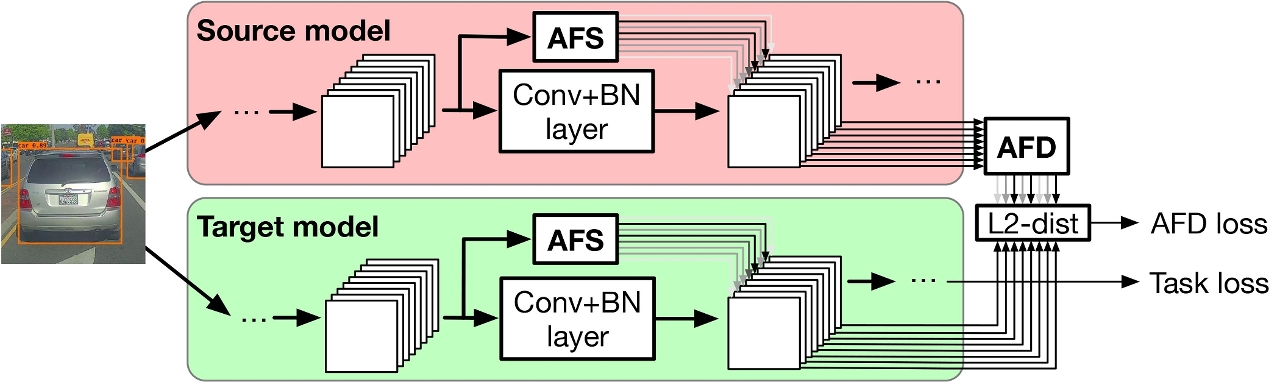
The University of Macau (UM) has achieved two major breakthroughs in autonomous driving research. A study at the university has provided new insights on autonomous driving under bad weather and complex road conditions while another study has developed new learning models that will be specifically applied to autonomous driving in Macao. The research results will be presented at two top annual conferences of artificial intelligence.
In recent years, the development of deep learning technology has greatly improved machine perception and machine’s cognition of the outside world. However, real world tasks often encounter problems when the test scene is different from the training scene. For example, researchers usually obtain abundant training data from self-driving vehicles on urban roads under standard driving conditions. However, if the trained model is directly applied under rare conditions, such as bad weather or bad road conditions, there often would be significant accuracy drops. To tackle this problem, a research project between UM and Baidu has proposed a method named RIFLE to periodically re-initialise the weight of a classifier in a learning mode. RIFLE has been proven to provide more meaningful gradient back-propagations. The researchers have also verified the effectiveness of RIFLE on various real-world transfer learning tasks, including several modern architectures (ResNet, Inception, MobileNet), and basic computer vision tasks (Classification, Detection, Segmentation). UM PhD student Li Xingjian is the first author of this paper. The paper has been accepted for presentation at 2020 International Conference on Machine Learning, a top conference in the field of artificial intelligence.
The second breakthrough comes from a study on the compression of CNN models in transfer learning. The study proposed a new compression method in which transfer and compression are used alternately. This method can reduce the complexity of the model and ensure high accuracy at the same time. By compressing part of the layer of the CNN model, the complexity can be further reduced. Experimental results verify that FLOPs of ResNet-101 can be reduced by 30 per cent on six target data sets, and the accuracy of compressed model remains almost unchanged. As FLOPs of the model are reduced by more than 90 per cent, the accuracy is maintained at around 0.70 on multiple data sets. In comparison, models generated by other methods basically failed to work. The paper has been accepted for presentation at the 2020 International Conference on Learning Representation. Wang Kafeng, a PhD candidate supervised by Prof Xu Chengzhong, is the first author of the paper. The study was jointly conducted by UM, the Shenzhen Institutes of Advanced Technology under the Chinese Academy of Sciences, and Baidu.
Macao Connected Autonomous Driving (MoCAD), led by UM’s State Key Laboratory of Internet of Things for Smart City, is funded by the Macao Funding Scheme for Key R&D Projects of the Science and Technology Development Fund, Macao SAR (File no. 0015/2019/AKP). The project aims to create a swarm intelligence based first-class vehicle platform and an experimental base of vehicle-road coordination for autonomous driving in the Guangdong-Hong Kong-Macao Greater Bay Area. It is a collaborative effort between UM and leading institutions in mainland China, including Shenzhen Institutes of Advanced Technology under the Chinese Academy of Sciences, the National University of Defense Technology, Baidu, and Shenzhen Haylion Technologies. The project is headed by Prof Xu Chengzhong, dean of UM’s Faculty of Science and Technology.